Researchers at EPFL have utilized deep reinforcement learning (DRL) to teach a robot how to adapt its gait to traverse complex terrains effectively, mimicking the movements of animals like trotting and pronking. Unlike previous beliefs that energy efficiency primarily drives gait transitions, this study highlights the significance of viability—or fall prevention—in motivating such adaptations.
The EPFL robot, employing DRL, learned to prioritize viability, adjusting its movement strategies to avoid falls while navigating terrains with gaps. Contrary to conventional wisdom, the study found that energy efficiency improvements are a result, rather than a cause, of gait transitions in challenging environments.
The research offers insights into the mechanisms behind gait transitions in animals. By focusing on viability, the robot dynamically adjusted its gait, switching from trotting to pronking when encountering gaps in the terrain to prevent falls. This prioritization of viability led to more agile robotic movement across uneven surfaces.
Using a bio-inspired learning architecture, the team trained the robot’s neural network to imitate the spinal cord’s transmission of brain signals, incorporating sensory feedback from the body. Through simulations, they observed that viability was the driving factor prompting gait transitions, highlighting its importance in locomotion control.
The researchers aim to further explore their findings by conducting experiments in various challenging environments, advancing our understanding of animal locomotion. Ultimately, they hope their work will contribute to the development of robots for biological research, reducing the reliance on animal models and addressing associated ethical concerns.
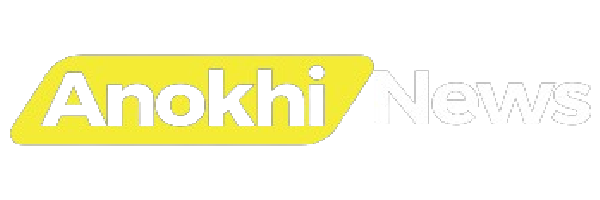