It’s like having a friend who gives different answers to the same question depending on how you ask it. If you ask, “What’s the capital of Peru?” you might get one response, but if you ask, “Is Lima the capital of Peru?” you might get a different one. That kind of inconsistency can make it hard to trust anything your friend says.
This inconsistency is exactly what’s happening with many large language models (LLMs), those super powerful AI tools like ChatGPT. A generative question, which is open-ended, might give one answer, while a discriminative question, which involves choosing between options, might give a different one. “There is a disconnect when the same question is phrased differently,” explained Athul Paul Jacob, a doctoral student at MIT.
To tackle this problem and make language models more reliable, Jacob and his team came up with a clever solution: a game called the consensus game. In this game, the model’s two modes are encouraged to find an answer they can agree on.
The game works like this: the generator, which handles generative questions, receives a question and some candidate responses. It then has to decide whether to answer correctly or incorrectly based on a coin toss. If it’s heads, it tries to answer correctly, and if it’s tails, it intentionally gives the wrong answer. The discriminator, which handles discriminative questions, then evaluates the response. Both sides are rewarded for reaching agreement and penalized for deviating too far from their original beliefs. Over many rounds of play, the two sides learn to align their responses and reach a consensus.
The results are promising. Language models that played the consensus game showed higher accuracy and internal consistency compared to those that didn’t. And the best part is, the game is computationally lightweight and doesn’t require any extra training or modification of the base model.
Jacob isn’t stopping there. He’s also exploring other ways to use game theory to improve language models, like a game called the ensemble game. With these innovative approaches, language models could become even better at handling complex interactions and providing reliable answers, no matter how the question is asked.
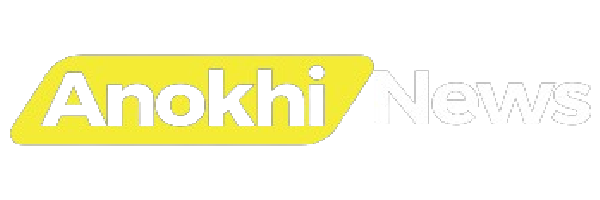